In recent years, the field of biology has undergone a transformative shift, driven by the advent of high-throughput technologies that generate vast amounts of data. From genomics and proteomics to imaging and metabolomics, the biological sciences are producing data at an unprecedented scale. This deluge of information, often referred to as “biological big data,” presents both an opportunity and a challenge. On one hand, it holds the promise of groundbreaking discoveries in areas such as personalized medicine, drug discovery, and understanding complex biological systems. On the other hand, the sheer volume and complexity of this data require advanced tools and methodologies for effective analysis. Enter artificial intelligence (AI), a powerful ally in the quest to unlock the secrets hidden within biological big data.
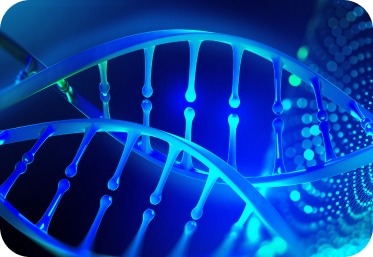
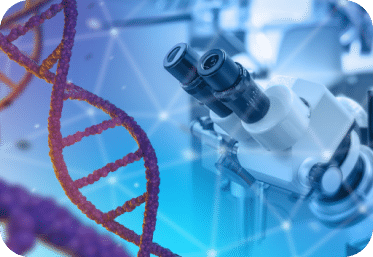
Understanding Biological Big Data
Before diving into the applications of AI, it’s essential to understand what constitutes biological big data. In the context of biology, big data encompasses a wide range of data types, including:
- 1. Genomic Data: Sequencing technologies like next-generation sequencing (NGS) generate massive amounts of genomic data, including DNA, RNA, and epigenetic information. These datasets are crucial for understanding genetic variations, disease mechanisms, and evolutionary processes.
- 2. Proteomic Data: Proteomics involves the large-scale study of proteins, including their structure, function, and interactions. Mass spectrometry and other high-throughput techniques produce vast amounts of proteomic data, which are essential for understanding cellular processes and disease pathways.
- 3. Imaging Data: Advanced imaging technologies, such as microscopy and medical imaging, generate high-resolution images of biological tissues, cells, and molecules. These images are rich in information but require sophisticated analysis techniques to extract meaningful insights.
- 4. Metabolomic Data: Metabolomics focuses on the comprehensive analysis of metabolites, the small molecules involved in cellular processes. This field generates large datasets that provide insights into metabolic pathways and their role in health and disease.
- 5. Clinical and Phenotypic Data: In addition to molecular data, biological big data includes clinical records, patient histories, and phenotypic information. Integrating these data types is critical for personalized medicine and understanding the genotype-phenotype relationship
The Role of AI in Biological Big Data Analysis
AI, particularly machine learning (ML) and deep learning (DL) techniques, has emerged as a powerful tool for analyzing biological big data. The application of AI in this domain can be categorized into several key areas:
- 1. Data Integration and Preprocessing: Biological data often come from diverse sources and in different formats. AI algorithms can be used to integrate these heterogeneous datasets, ensuring that the information is consistent and ready for analysis. AI-driven data preprocessing techniques, such as dimensionality reduction and normalization, help manage the complexity and scale of the data.
- 2. Pattern Recognition and Feature Extraction: AI excels at recognizing patterns in complex datasets, making it invaluable for tasks such as identifying biomarkers, classifying disease states, and predicting outcomes. For example, deep learning models can automatically extract features from genomic or imaging data, enabling the discovery of novel biological insights without the need for manual feature selection.
- 3. Predictive Modeling and Classification: One of the most significant contributions of AI to biological big data analysis is its ability to build predictive models. These models can be used to predict disease risk, patient outcomes, or drug responses based on genomic and clinical data. AI-based classifiers are also widely used in tasks such as cancer diagnosis, where they can distinguish between different types of tumors with high accuracy.
- 4. Network Analysis and Systems Biology: Biological systems are highly interconnected, with complex networks of genes, proteins, and metabolites interacting in intricate ways. AI techniques, particularly those based on graph theory and network analysis, can be used to model these interactions and identify key regulatory elements, such as hubs or bottlenecks in biological networks
- 5. Drug Discovery and Development: The pharmaceutical industry is increasingly leveraging AI to accelerate drug discovery and development. AI algorithms can analyze large-scale chemical, genomic, and clinical data to identify potential drug candidates, predict their efficacy, and optimize their design. This approach significantly reduces the time and cost associated with bringing new drugs to market.
- 6. Personalized Medicine: Personalized medicine aims to tailor medical treatment to the individual characteristics of each patient. AI plays a crucial role in this field by analyzing genomic, proteomic, and clinical data to identify personalized treatment strategies. For example, AI-driven models can predict how a patient will respond to a particular drug, enabling more effective and targeted therapies.
- 7. Interpretation and Visualization: The complexity of biological big data necessitates advanced methods for interpretation and visualization. AI-driven tools can transform raw data into intuitive visual representations, such as heatmaps, networks, and 3D models. These visualizations aid researchers in understanding complex biological phenomena and communicating their findings.
Challenges and Future Directions
While AI has made significant strides in biological big data analysis, several challenges remain. One of the primary challenges is the need for large, high-quality datasets to train AI models effectively. Additionally, the “black box” nature of some AI algorithms, particularly deep learning models, can make it difficult to interpret their predictions and understand the underlying biological mechanisms.
Looking forward, the integration of AI with other emerging technologies, such as quantum computing and synthetic biology, holds the potential to further revolutionize biological research. Moreover, as AI algorithms become more transparent and interpretable, their adoption in clinical settings is likely to increase, paving the way for more widespread use in personalized medicine.
Conclusion
The application of AI in biological big data analysis is transforming the way we approach complex biological questions. By harnessing the power of AI, researchers can make sense of vast and intricate datasets, uncovering new insights into the mechanisms of life and disease. As AI continues to evolve, its role in biology will only grow, driving innovations that have the potential to improve human health and our understanding of the natural world.